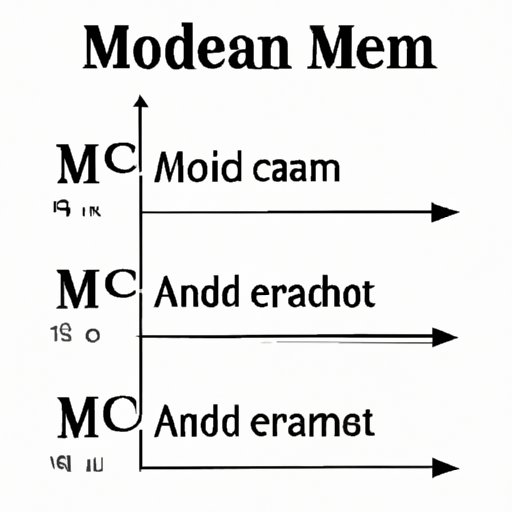
I. Introduction
Data analysis requires various mathematical tools to interpret and make meaningful insights from datasets. One such tool is a measure of central tendency, which summarizes data to provide a typical or representative value. This article focuses on the median, a commonly used measure of central tendency. The median is a valuable method for analyzing datasets and is particularly useful in outlier and skewed data situations. In this article, we will explore the importance of finding the median, how to calculate it and how to interpret it.
A. Explanation of the problem
Given a dataset, how do we find the value that best represents that dataset?
B. Importance of finding the median
The median is an important measure of central tendency because it provides a representative value that is less affected by outliers and skewed data than other measures such as the mean. For instance, if a dataset contains extreme values, like a per-person income with a few billionaires in the mix, the mean would not provide an accurate representation of the typical income. The median, by definition, represents the middle point of the data set and would not be affected by those few billionaires. In this way, the median is a more robust measure and a critical tool in data analysis.
C. Overview of the article structure
This article will cover various aspects of median, including how to calculate it, how to interpret it, and how to apply it in real-world data analysis. Additionally, we provide a simplified step-by-step guide for beginners to find and calculate the median with ease. Combined, these approaches will provide a comprehensive understanding of the median and its importance in data analysis.
II. “5 Simple Steps: How to Find the Median of a Data Set”
Let’s start with a straightforward step-by-step approach for finding the median of a dataset:
A. Step 1: Sorting the data in ascending/descending order
To find the median, we need to sort the data set in either ascending or descending order.
This process helps us identify the middle position(s) in the data set.
B. Step 2: Determining the number of data points
Next, we need to count how many data points are in the dataset. This step is critical as it helps us determine if the number of data points is even or odd.
C. Step 3: Identifying if the number of data points is even/odd
If the number of data points is odd, there is a single value that corresponds to the middle of the data set, and that value represents the median. If the number of data points is even, there are two values that correspond to the middle of the data set.
D. Step 4: Finding the middle data point/points
For an odd number of data points, find the ‘middle’ position by counting half-way through the data set, starting from the left. For instance, if there are nine data points, the median would be the fifth value. If there are 15 data points, the median would be the eighth value in the sorted dataset. If there is an even number of data points, the median is the average of the two middle data points.
E. Step 5: Calculating the median
After identifying the middle data points, the final step is to calculate the median. If there is a single middle value, that value is the median. If there are two middle values, the median is the average of the two middle values. For example, if the sorted list is {1, 2, 3, 4, 5, 6, 7, 8, 9} then the median would be 5 as count halfway through.
III. “Mastering the Median: A Beginner’s Guide”
A. Definition and explanation of the median
In statistics, the median is a common measure of central tendency. The median represents the middle point in a dataset after the data points have been sorted from smallest to largest. The median is not influenced by extreme values and outliers, making it a more robust measure than the arithmetic mean. Median gives more importance to the middle values and downplays the outliers as they don’t cause any significant change in its value.
B. Advantages of using the median
One of the main advantages of the median is its robustness. The median is not affected by outliers or extreme values in the data set and can provide a more accurate representation of the typical value when compared to the central tendency measure like mean. The median is easy to calculate and is less sensitive to small changes in the data set.
C. Comparison of the median with other measures of central tendency
It is vital to understand the difference between the median and other measures of central tendency like mode and mean. The mean is the sum of all data points in the data set divided by the number of data points. The median is the middle value of a dataset. The mode is the data point that occurs most frequently in the dataset. The mean is affected by outliers and skewed data, making the median a more robust measure of central tendency in such cases. The mode is the most common data point but is not as useful when the data set is not smooth. Median gives a better representation of the data sets’ central value when compared to mode and mean.
IV. “Exploring the Middle Ground: Finding the Median in Statistics”
A. Explanation of median in statistics
Median is a measure of central tendency that provides the middle value in a dataset. It separates the data set into two halves – the lower half and the upper half. The median is a valuable tool in statistics because it is not as affected by outliers or skewed data as the mean. Median gives more significance to the middle values and less emphasis on the outliers or the extreme values which may have the effect of causing a wrong conclusion.
B. Calculation of median in different types of data distributions
Median calculation may vary depending on the type of data distribution. For example, finding the median in a skewed right data distribution is relatively straightforward: it is the point, that is one-half of the way between the median and the highest number. In contrast, finding the median in a skewed left data distribution is more complicated: it is the point that is one-half of the way between the median and the smallest number.
C. Role of median in statistical analysis
The median is particularly useful in statistical analysis because it helps in drawing insights and conclusions from datasets. It is a crucial measure of central tendency when dealing with data sets that include outliers, skewed data or are not normally distributed. Median helps us to understand the central location of the dataset, making it a vital tool for researchers to make inferences and predictions from their findings.
V. “From Highs to Lows: Understanding the Median in Data Analysis”
A. Identifying outliers and skewed data
Outliers, extreme values, and data sets with an asymmetric distribution are common in real-world data analysis. They can negatively impact our results and conclusions by skewing our measurements of central tendency. Identifying these values and data sets’ skewed distribution is an important first step before calculating the median.
B. Role of median in handling outliers
Median is an excellent tool to handle outliers because it is not influenced by their extreme values, unlike the mean. When the data set contains outliers, the median is more robust in representing the center of the dataset. It helps to view both extremes, allowing researchers to make unbiased observations and conclusions.
C. Limitations of median in skewed data
In skewed data, the median may not provide an accurate representation of the data set, especially when it is used alone. In this case, it is advisable to supplement it with additional statistical measures, such as mode and quartiles.
VI. “Back to Basics: How to calculate the Median of a Data Set with ease”
A. Simplified method for finding the median
To find the median of a dataset, follow these steps:
- Sort the data set in ascending or descending order
- Determine the number of data points
- If the number of data points is odd, the middle value is the median. If the number of data points is even, average the two middle values.
B. Example problem for practice
Suppose you have a data set of {2, 4, 6, 8, 10, 12, 14, 16, 18}. Find the median.
Step 1: Sort the dataset in ascending order:
2, 4, 6, 8, 10, 12, 14, 16, 18
Step 2: Determine the number of data points (n) = 9
Step 3: Identify the middle value by counting halfway through the dataset
The middle value is 10. Therefore, the median of the data set is 10.

VII. “The Power of the Median: Unlocking Insights in Your Data”
A. Importance of median in real-world data analysis
The importance of median in real-world data analysis cannot be overstated. It provides insights into the center of the data set that cannot be provided by other measures of central tendency. Median is commonly used in various fields, including economics, healthcare, and sociology, among others, to summarize data and make predictions.
B. Interpretation of median in different fields
The interpretation of median varies depending on the field of study. For example, in healthcare, median is a useful tool in analyzing patient data, such as hospital stays and medication effectiveness. In economic studies, median is significant in analyzing income and unemployment rates. The interpretation of median varies depending on the study objective, and the data being analyzed.
C. Examples of median in action
An example of median in action can be seen in analyzing the salaries of individuals. In such cases, finding the median will provide the typical salary, unlike the mean, which may be inflated due to the inclusion of high paying individuals. This information helps governments and organizations in making informed policy and business decisions.
VIII. “Finding Balance: Discovering the Median in Skewed Data Sets”
A. Challenges in finding median in skewed data
Finding median in skewed data can be challenging because the median may not be an appropriate representation of the data set. In some situations, the median may be significantly higher or lower than the preponderance of data and may not be the best method. In such situations, using other measures of central tendency, including mode and quartiles, may provide a better representation of the dataset.
B. Techniques for handling skewed data in median calculation
Several techniques can handle skewed data in median calculation. The most common include using logarithmic scales, transformation methods, and nonparametric methods.
C. Real-world examples
An excellent example of skewed data can be seen in wealth distribution in many countries. Wealth distribution data tends to be right-skewed, and the median may not provide an accurate representation of the data set. In such cases, the Gini coefficient, which measures inequality, and the coefficient of variation, which measures dispersion, may provide a more significant representation of the data set.
IX. Conclusion
A. Recap of the importance of the median
This article explored the median as a measure of central tendency. It is an important and widely used tool for data analysts because it is more robust than other measures like mean and mode. The median is particularly useful in handling outliers and skewed data, where other measures may not provide an accurate representation of the dataset.
B. Encouragement to apply the learning
It is vital to know when and how to use the median accurately to draw meaningful insights. With the simplified method and examples provided in this article, you can now calculate and interpret the median with ease.
C. Next steps for further study
For individuals wanting more complex methods and in-depth understanding of median, further study is necessary. They can explore other statistical methods and tools such as the interquartile range and percentile.