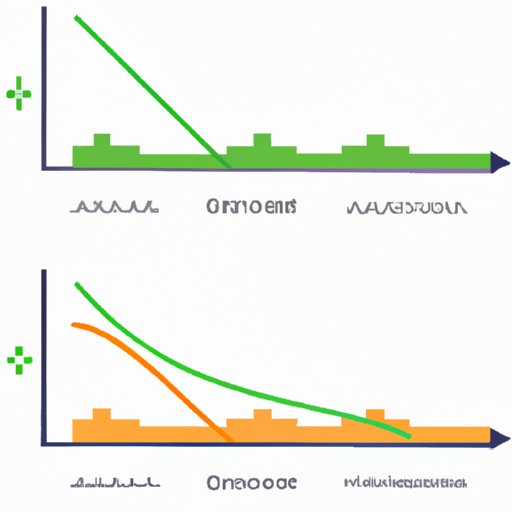
I. Introduction
Regression analysis is a statistical technique that helps to identify the relationship between a dependent variable and one or more independent variables. This technique plays a critical role in data-driven decision-making in different industries such as finance, healthcare, and marketing. Since its introduction, regression analysis has undergone significant improvements and advancements that have made it a valuable tool for businesses. This article will serve as a beginner’s guide to regression analysis, highlighting its basics, importance, real-life applications, and future trends.
II. A Beginner’s Guide to Regression Analysis: Understanding the Basics and How to Get Started
Regression analysis has two types: simple and multiple. A simple regression analysis involves only one independent variable, while a multiple regression analysis involves two or more independent variables. The basic concept of regression analysis is to identify the relationship between the independent variable(s) and the dependent variable. The purpose of regression analysis is to develop a mathematical model that can be used to predict the value of the dependent variable based on the value(s) of the independent variable(s).
Before conducting regression analysis, it is essential to understand its assumptions. These assumptions include linear relationships, independence of errors, homoscedasticity, and normality. Understanding these assumptions is key to ensuring that regression analysis is done correctly and effectively.
Performing simple regression analysis can be done using software tools like R or Excel. These software tools have built-in regression analysis functionalities that make it easy for beginners to use. The following are the steps to perform simple regression analysis using Excel:
- Enter the data into Excel, with the dependent variable in one column and the independent variable in another column.
- Select the “Data” tab in the Excel Ribbon and click on “Data Analysis.”
- Select “Regression” from the list of available analysis tools and click on “OK.”
- Enter the input and output ranges, selecting the column that contains the dependent variable as the output variable and the column that contains the independent variable as the input variable.
- Click on “OK” to generate the output.
III. Why Regression Analysis is Critical for Data-Driven Decision Making and How to Do It Right
Regression analysis is critical for business decision-making because it helps to identify the significant factors that influence outcomes. It also helps in predicting future outcomes, which is essential in making informed decisions. Understanding how to do regression analysis correctly is crucial in ensuring that the results obtained are reliable. One common mistake is violating the assumptions of regression analysis. Other mistakes include overfitting, multicollinearity, and ignoring outliers.
To do regression analysis right, there are tips that businesses can apply. These tips include using cross-validation to avoid overfitting, assessing multicollinearity, examining influential points, and using appropriate measures of fit.
IV. Regression Analysis vs. Correlation: What’s the Difference and How to Use Each Effectively
Regression analysis and correlation are both statistical techniques used to measure the relationship between variables. The key difference between the two is that regression analysis seeks to establish a cause-and-effect relationship, while correlation only measures the strength of the relationship. Correlation can be positive or negative, depending on the direction of the relationship.
One instance where correlation would be preferred over regression analysis is when the focus is only on measuring the strength of the relationship between variables. Regression analysis should be used when there is a need to establish a cause-and-effect relationship and identify the significant factors that influence outcomes.
The strengths of correlation and regression analysis include their ability to identify the relationship between variables and predict outcomes. However, the limitations of correlation and regression analysis include the assumption of linearity, the presence of outliers, and the impact of extraneous variables.
V. Regression Analysis in Real Life: How Businesses Are Leveraging It to Optimize Outcomes
Regression analysis has several real-life applications in different industries. For instance, in the finance industry, businesses use regression analysis to identify the factors that influence stock prices, interest rates, and bond yields. In healthcare, regression analysis is used to examine the factors that determine medical treatment outcomes and patient satisfaction. In marketing, regression analysis helps businesses understand customer behavior and develop effective advertising strategies.
While regression analysis is a useful tool, businesses may face challenges when using it. For example, data collection can be time-consuming and expensive. Additionally, businesses may need to have a clear understanding of the relationships between the variables they are analyzing to use regression analysis effectively.
VI. The Future of Regression Analysis: Innovations and Trends to Keep an Eye On
The field of regression analysis is continuously evolving to meet the changing needs of businesses. One innovation that will impact regression analysis is machine learning. Machine learning algorithms enable the use of advanced techniques like artificial neural networks, boosted trees, and support vector machines to conduct regression analysis. Other trends worth noting include the increased use of big data, the incorporation of data visualization, and the use of automated reporting.
Businesses that want to stay up-to-date with the latest trends in regression analysis should consider investing in training programs and advanced software tools. They can also hire professionals with experience in regression analysis to provide insights and help them leverage its benefits effectively.
VII. Conclusion
Regression analysis is a powerful statistical technique used to measure the relationship between variables. Its importance in data-driven decision-making cannot be overstated, as it helps businesses predict future outcomes, identify significant factors that influence outcomes, and develop effective strategies. By understanding the basics, importance, and real-life applications of regression analysis, businesses can leverage its benefits effectively and stay ahead of the competition.
Readers are encouraged to apply the knowledge gained from this article into their work to make informed decisions informed by Regression Analysis.