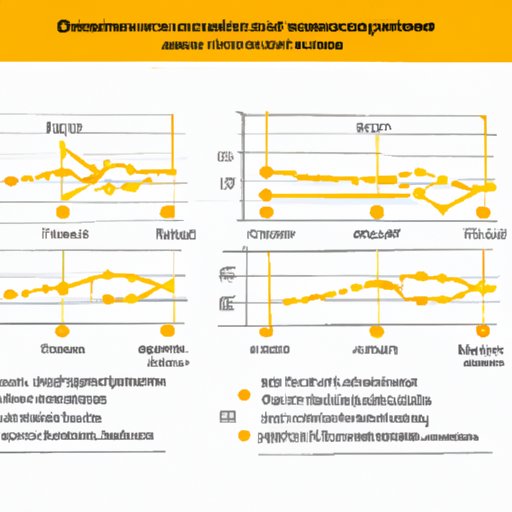
Introduction
Confidence intervals are an essential tool in data analysis. They provide important information about the accuracy of statistical estimates and can help individuals make informed decisions based on their data. In this article, we will explore the definition of confidence intervals, the role they play in statistical inference, and step-by-step guides for calculating them.
The Importance of Confidence Intervals: An In-Depth Explanation for Beginners
Confidence intervals play a significant role in statistical inference. They provide a range of values in which a population parameter is likely to fall, based on a sample of data. Having this information gives us confidence in the accuracy of our statistical estimates and can inform decision-making. For instance, a pharmaceutical company may use confidence intervals in drug trials to determine if a new drug is effective.
Confidence intervals provide essential information about the accuracy of our statistical estimates. They tell us how much uncertainty there is in our data, and we can use this information to make more informed decisions. For example, if a confidence interval is narrow, we can be more confident that our statistical estimate is accurate. On the other hand, if our confidence interval is wide, we may need to collect more data to get a more precise estimate.
Walkthrough Guide: A Step-by-Step Approach to Calculating Confidence Intervals
Before we dive into calculating confidence intervals, it’s essential to understand some key terms. The following are definitions of some crucial concepts you’ll need to know:
- Sample mean: The arithmetic mean of a sample
- Standard deviation: A measure of how much variation there is in a sample or population
- Population mean: The average value of a population
- Confidence level: The likelihood that a confidence interval will contain the true population parameter
- Z-score: The number of standard deviations from the mean of a normal distribution
Now, let’s go through a step-by-step guide to calculate confidence intervals:
- Determine the sample mean: Calculate the arithmetic mean of your sample data
- Determine the standard deviation: Calculate the standard deviation of your sample data
- Determine the sample size: Calculate the sample size or number of observations in your sample
- Determine the confidence level: Choose a confidence level, usually 90%, 95%, or 99%.
- Determine the z-score: Based on your confidence level, look up the appropriate z-score from a standard normal distribution table
- Calculate the margin of error: Multiply the z-score by the standard deviation, then divide by the square root of the sample size
- Calculate the lower and upper limits of the confidence interval: Subtract the margin of error from the sample mean to get the lower limit, and add the margin of error to the sample mean to get the upper limit.
For example, let’s calculate a 95% confidence interval for a sample with a mean of 10, a standard deviation of 2, and a sample size of 50. The appropriate z-score for 95% confidence level is 1.96. Multiplying 1.96 by the standard deviation (2) and dividing by the square root of the sample size (50) gives a margin of error of 0.56. Therefore, the lower limit of the confidence interval is 9.44 (10 – 0.56), and the upper limit is 10.56 (10 + 0.56).
Mastering Confidence Intervals: How to Determine Intervals with Ease
Choosing the appropriate confidence level and sample size is crucial in determining the precision of your statistical estimate. A confidence level of 95% is often used because it provides a good balance between accuracy and practicality. You also need to ensure that your sample size is not too small and not too large. A sample that is too small may not accurately represent the population, while a sample that is too large may be too costly to collect.
Calculating confidence intervals can be a time-consuming and confusing process, but there are things you can do to make it easier. Here are some tips for simplifying the process of calculating confidence intervals:
- Use statistical software to calculate confidence intervals
- Use online calculators to automate the process
- Use tables of z-scores to find critical values quickly
Simplifying Statistics: Your Complete Guide to Calculating Confidence Intervals
There are some common misconceptions about confidence intervals. One is that a confidence interval tells us the probability that the true population parameter falls within that range. However, a confidence interval only tells us the likelihood that a range contains the true population parameter, given our sample data and confidence level. Another misconception is that a wider confidence interval means that the sample data is less accurate. However, a wider confidence interval could mean that our confidence level is lower, or our sample size is smaller.
Strategies for simplifying complex calculations include utilizing software or online calculators. Another option is to use tables of critical values to find z-scores quickly. You can also practice calculating confidence intervals with sample problems to improve your skills.
Mathematical Approach: Equations and Formulas for Calculating Confidence Intervals
If you prefer to calculate confidence intervals mathematically, there are specific equations and formulas that you’ll need to know:
- For a confidence interval of a population mean with known variance: (sample mean) +/- (z-score) * (standard error)
- For a confidence interval of a population mean with unknown variance: (sample mean) +/- (t-score) * (standard error)
- For a confidence interval of a proportion: (proportion) +/- (z-score) * (standard error)
Where the standard error is calculated as the standard deviation divided by the square root of the sample size.
For example, if we want to calculate a 95% confidence interval for a population mean with unknown variance, we first calculate the t-score based on the sample size and confidence level. Suppose we have a sample size of 100 and a confidence level of 95%. In that case, our t-score is 1.98. If our sample mean is 8 and our standard deviation is 2, our margin of error is 0.4. So our confidence interval is (7.6, 8.4).
Real-World Application: Understanding How and Why Confidence Intervals are Used in Data Analysis
Confidence intervals are used in various real-world situations to make informed decisions. For example, a marketing research company may use confidence intervals to determine the proportion of customers who prefer a particular product. A transportation company may use confidence intervals to estimate the number of buses that will be needed to transport passengers on a busy holiday weekend. A hospital may use confidence intervals to determine the effectiveness of a treatment.
Interpreting confidence intervals can inform decision-making in critical situations. If a confidence interval for a drug’s effectiveness is too wide, a pharmaceutical company may need to consider collecting more data or reevaluating the trial’s results. On the other hand, if a confidence interval for a market research study’s results is very narrow, a company may decide to invest in a new product based on the results.
Conclusion
Calculating confidence intervals is an essential skill for anyone involved in data analysis. By using the appropriate statistics, understanding the key terms involved, and following a step-by-step process, you can calculate accurate confidence intervals that can inform decision-making. Confidence intervals are used in many real-world applications, from medical research to market research, and understanding their significance is crucial. By mastering confidence intervals, you can enhance your data analysis skills and make more informed decisions.