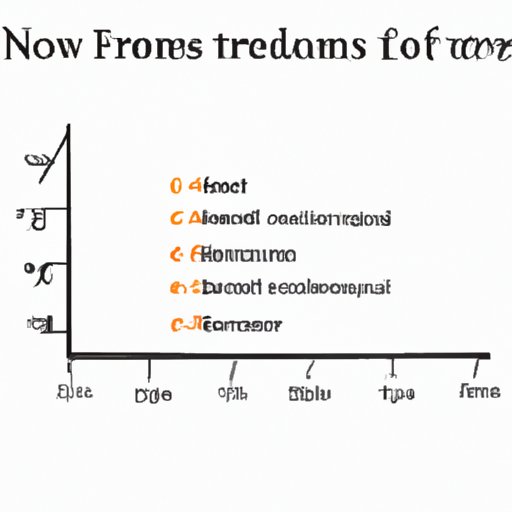
Introduction
Statistics involves conducting experiments to draw conclusions that inform decision making. However, the results of statistical experiments can never be wholly accurate due to inherent errors in sampling, measurement, and variation. A statistical experiment’s success is subject to randomness and degrees of freedom play an indispensable role in quantifying such errors.
A degree of freedom is a statistical concept that relates to the estimation of population parameters based on sample data. The use of degrees of freedom is necessary when creating confidence intervals or performing hypothesis testing to calculate the variability of the estimates drawn from the sample. Therefore, grounding an understanding of degrees of freedom is crucial for quantitative researchers, analysts, and scientists globally.
This article delves into the fundamental concepts behind degrees of freedom and the importance of their calculation in statistical analysis.
Degrees of Freedom Demystified: A Step-by-Step Guide to Calculating Them
Degrees of freedom refer to the number of data elements within a statistical calculation that contain useful information for drawing valid statistical inferences. In a nutshell, the degrees of freedom are used to calculate sample statistics such as the variance, standard deviation, or the mean by defining the number of data points that are free to vary. Simply put, more degrees of freedom translate to more reliable sample statistics.
Calculating degrees of freedom can be confusing and frustrating. However, it can be demystified through systematic steps:
- Step 1: Identify the sample size and the parameters being estimated.
- Step 2: Subtract one from the sample size to obtain the number of degrees of freedom for estimating the sample variance or standard deviation. For instance, if the sample size is twenty, the degrees of freedom for variance will be 19, and while estimating the standard deviation, the degrees of freedom will be 18;
- Step 3: Subtract the number of parameters being estimated from the degrees of freedom obtained. Parameters are values that are initially unknown but are to be estimated to draw meaningful conclusions. For instance, when comparing the means of two groups, one parameter needs to be estimated. Therefore, the degrees of freedom would be 19-1=18.
Why Knowing Degrees of Freedom is Crucial in Statistical Analysis: A Beginner’s Guide
Degrees of freedom play a fundamental role in statistical experimentation as they are used to test hypotheses and conduct significance tests. Significance tests are instrumental in determining whether an intervention has a statistically significant impact, making them fundamental in making informed decisions. The knowledge of degrees of freedom is crucial in conducting such tests and obtaining valuable results.
There are several common misconceptions that researchers have regarding the calculation and importance of degrees of freedom. For instance, some believe that degrees of freedom refer to the total amount of data points in a dataset or experiment. While it’s crucial to consider the total amount of data points, this is not the basis of the calculation of degrees of freedom.
Another misconception is that degrees of freedom determine the statistical significance of the test. While the degrees of freedom help determine the variability of the estimates, they do not give information regarding the statistical significance of the results.
Degrees of freedom do not merely play a pivotal role in statistical analysis. They are important in other disciplines, including natural and social sciences, engineering, and even economics.
The Importance of Degrees of Freedom in Research: How to Calculate Them with Examples
Degrees of freedom are necessary for many statistical tests, including t-tests, ANOVAs and chi-square tests. The calculation of degrees of freedom depends on the statistical test being conducted and the parameters being estimated.
The degrees of freedom calculation plays a crucial role in significance testing and hypothesis testing. In hypothesis testing, degrees of freedom are used to estimate the probability of obtaining particular test results when the null hypothesis is true.
The accurate calculation of the degrees of freedom is critical in ensuring that research results are reliable and valid. Failure to calculate the degrees of freedom accurately may lead to incorrect or biased results, leading to erroneous conclusions.
Let’s consider an example where we want to determine if there is any difference between the mean height of a particular population sample group and another one. Here, we would have two mean values that need to be estimated, hence one degree of freedom is lost.
How to Calculate Degrees of Freedom for T-tests, ANOVAs, and Other Statistical Tests
The calculation of degrees of freedom varies depending on the test being conducted. The following are some of the most common statistical tests and how to calculate their degrees of freedom:
T-tests
T-tests are useful in comparing the means of two different samples. Degrees of freedom, in this case, are calculated as the sum of the sample sizes minus two.
ANOVAs
ANOVA (Analysis of Variance) involves comparing data across three or more groups. The degrees of freedom are calculated by subtracting one from the total number of samples.
Chi-Square
The chi-square test is used to analyze nominal and categorical variable data and involves calculating how well an observed distribution matches an expected distribution. For a chi-square test, the degrees of freedom equation is (rows – 1) * (columns – 1).
Unlocking the Mystery of Degrees of Freedom: Simple Tips for Accurate Calculations
The most basic rule of the accurate calculation of degrees of freedom is to ensure that the estimator’s constraint is not violated. This is often the case when the sample size is too small, resulting in degrees of freedom being negative or zero.
Avoid using an observed distribution’s values to calculate degrees of freedom, as this may result in bias and incorrect results.
Finally, while there are set formulas to calculate degrees of freedom, some calculations may need to be approximated, especially in cases when sample sizes are large.
Conclusion
This article has explored the concept of degrees of freedom and its importance in statistical analysis. Understanding degrees of freedom is essential to calculate confidence intervals and conducting hypothesis and significance tests accurately.
Additionally, the article has clarified common misconceptions around the calculation and significance of degrees of freedom. The calculation of degrees of freedom is vital in numerous statistical tests, including ANOVAs, chi-square, and t-tests.
As a beginner, understanding the steps to calculate the degrees of freedom helps reduce confusion and ensure accurate results for statistical tests.
Finally, the article has provided tips and tricks that help in the accurate calculation of degrees of freedom. For instance, ensuring that estimator constraints are not violated and approximating results to make calculations more feasible.
With the comprehensive knowledge and the tips provided in this article, you will confidently apply it in your statistical analysis practices, making meaningful conclusions.