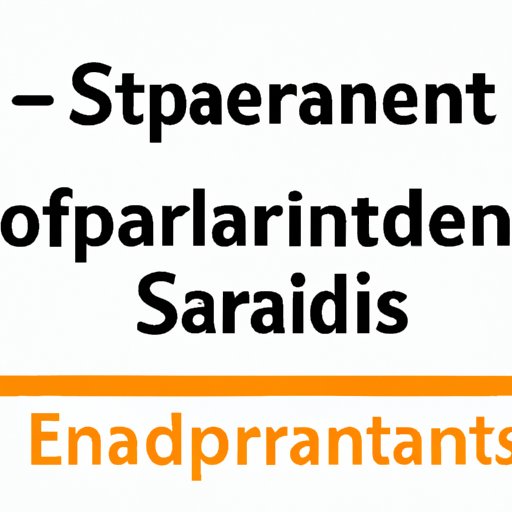
I. Introduction
Standard error is an important concept in statistical analysis that helps us understand the variability in our data. It’s essential to have a good grasp of this concept when working with data, particularly when making inferences about populations based on samples.
In this article, we’ll delve into the details of how to calculate standard errors. We’ll start with the basics and progress to more advanced techniques, providing a step-by-step guide for beginners along the way.
A. Explanation of what standard error is
Standard error is the standard deviation of the sampling distribution of a statistic. It measures the variability of a statistic such as the sample mean or proportion and tells us how much we can expect that statistic to vary from sample to sample due to chance alone. The larger the standard error, the more uncertain we are about the estimated value of the statistic.
B. Importance of standard error in statistical analysis
Standard error is a crucial tool for statistical analysis. It allows us to estimate the precision of our estimates and make inferences about populations based on samples. Without a good understanding of standard error, it’s easy to misinterpret our data and draw inappropriate conclusions.
C. Brief overview of the topics that will be covered
In the following sections, we’ll cover the basics of standard error calculation, common mistakes to avoid, the relationship between standard error and confidence intervals, tips for improving your calculations, and advanced strategies for use with large datasets. By the end of this article, you’ll have a comprehensive understanding of standard error and how to calculate it accurately.
II. Mastering Standard Error Calculations: A Step-by-Step Guide for Beginners
A. Explanation of basic concepts and terminology
Before we jump into the calculations, let’s review some basic concepts and terminology. First, we need to distinguish between the population and the sample. The population is the entire group of individuals or objects that we’re interested in studying, while the sample is the subset of the population that we actually observe or measure.
Next, we need to understand the concept of a statistic. A statistic is a numerical summary of the data, such as the mean, median, or standard deviation. We use statistics to estimate population parameters, such as the population mean or standard deviation.
The standard error is a measure of the variability of a statistic due to chance. It represents the standard deviation of the sampling distribution of the statistic. The sampling distribution is the distribution of all possible values of the statistic that we could obtain from all possible samples of a given size from the population.
B. Step-by-step guide to calculate standard error with a simple example
Now let’s put these concepts into practice with a step-by-step guide to calculate standard error. Suppose we want to estimate the population mean height of adult males in the US. We take a random sample of 100 adult males and measure their heights. The sample mean height is 70 inches, and the sample standard deviation is 3 inches.
1. Determine the sample size, n.
2. Calculate the sample mean, x̄.
3. Calculate the sample standard deviation, s.
4. Calculate the standard error, SE.
Using our example:
1. n = 100
2. x̄ = 70 inches
3. s = 3 inches
4. SE = s / √n = 3 / √100 = 0.3 inches
So the standard error of the sample mean height is 0.3 inches. This tells us how much we can expect the sample mean to vary from sample to sample due to chance alone.
C. Common mistakes to avoid
When calculating standard error, there are several common mistakes to avoid. One of the most common mistakes is to confuse standard error with standard deviation. Remember that standard deviation measures the variability of the data, while standard error measures the variability of the sampling distribution of a statistic.
Another common mistake is to use the wrong formula for standard error. Remember that the formula for standard error depends on the statistic you’re interested in estimating and the sampling distribution of that statistic. Be sure to use the correct formula for your particular situation.
A final common mistake is to assume that the population is normally distributed when it may not be. Remember that the central limit theorem tells us that the sampling distribution of certain statistics, such as the sample mean, will be approximately normal as long as the sample size is large enough. However, if the population distribution is highly skewed or has outliers, the sampling distribution may not be normal. In these cases, alternative methods such as bootstrapping may be necessary.
III. The Importance of Standard Error and How to Calculate It
A. Why standard error is important
Standard error is important because it allows us to estimate the precision of our estimates and make inferences about populations based on samples. Suppose we want to estimate the population mean income of US households. We take a random sample of 1000 households and calculate the sample mean income. Without knowing the standard error of the sample mean, we have no way of knowing how certain we are about our estimate. A large standard error would indicate that our estimate is uncertain and subject to a high amount of variation from sample to sample due to chance.
B. Different methods to calculate standard error
There are several methods to calculate standard error, depending on the statistic you’re interested in estimating and the sampling distribution of that statistic. Some common methods include:
- Using the sample standard deviation, s, and the sample size, n, to estimate the standard error of the sample mean.
- Using the sample proportion, p, and the sample size, n, to estimate the standard error of the sample proportion.
- Using the regression standard error to estimate the standard error of the regression coefficients in linear regression.
C. Real-world applications of standard error
Standard error has many real-world applications in fields such as economics, epidemiology, and social science. For example, in economics, standard error is used to estimate the precision of estimates of the effects of policies or interventions on outcomes such as income, education, and health. In epidemiology, standard error is used to estimate the precision of estimates of disease prevalence or incidence rates. In social science, standard error is used to estimate the precision of estimates of opinions or attitudes in surveys or polls.
IV. Errors and Confidence Intervals: Understanding the Science Behind Standard Error Calculations
A. Explanation of sampling distribution of a statistic
The sampling distribution of a statistic is the distribution of all possible values of the statistic that we could obtain from all possible samples of a given size from the population. The shape of the sampling distribution depends on the population distribution, the sample size, and the statistic of interest.
B. How standard error is related to confidence intervals
Standard error is used to calculate confidence intervals, which are a range of values that are likely to contain the true value of the population parameter with a certain level of confidence. For example, a 95% confidence interval for the population mean of a variable will contain the true population mean with 95% confidence. The formula for the confidence interval depends on the sampling distribution of the statistic and the desired level of confidence.
C. Calculating confidence intervals with standard error
To calculate a confidence interval with standard error, we use the following formula:
Lower bound = point estimate – z*SE
Upper bound = point estimate + z*SE
where point estimate is the statistic we’re interested in estimating, SE is the standard error of that statistic, and z is the critical value from the standard normal distribution corresponding to the desired level of confidence. For a 95% confidence interval, z = 1.96.
V. Improving Your Data Analysis: Tips and Tricks for Accurate Standard Error Calculations
A. Common mistakes to avoid when calculating standard error
Some common mistakes to avoid when calculating standard error include:
- Using the wrong formula for the statistic you’re interested in estimating
- Confusing standard error with standard deviation
- Assuming normality of the population when it may not be appropriate
B. Ways to increase accuracy, such as increasing sample size
There are several ways to increase the accuracy of standard error calculations, such as:
- Increasing the sample size
- Using more precise measurement tools
- Reducing measurement error
Increasing the sample size is particularly effective in reducing the standard error of the sample mean. As the sample size increases, the standard error approaches zero, assuming that the population variance is fixed.
C. Examples of how improved standard error calculations can affect statistical analysis
Improved standard error calculations can have a significant impact on statistical analysis. For example, suppose we want to estimate the effect of a new drug on blood pressure. We conduct a randomized controlled trial with two treatment groups and a control group. We measure blood pressure at baseline and after treatment. We calculate the mean change in blood pressure for each group and conduct a hypothesis test to determine if there is a statistically significant difference between the treatment groups and the control group. If our standard error calculations are inaccurate, we may draw incorrect conclusions about the effectiveness of the drug or miss important treatment effects.
VI. Going Beyond the Basics: Advanced Strategies for Calculating Standard Errors with Large Datasets
A. Explanation of bootstrapping and robust standard errors
Bootstrapping is a resampling technique that involves repeatedly sampling from the original sample to estimate the sampling distribution of a statistic. This approach allows us to estimate standard errors without making assumptions about the shape of the population distribution or the sampling distribution of the statistic. Robust standard errors are a related technique that involves adjusting standard errors to account for violations of assumptions such as non-normality or heteroscedasticity.
B. Advantages and disadvantages of these methods
The main advantage of bootstrapping and robust standard errors is their flexibility in dealing with real-world data scenarios that may violate assumptions of traditional statistical methods. However, these methods can be computationally intensive and may require larger sample sizes to achieve reliable estimates compared to traditional methods.
C. How to apply these methods with large datasets
With large datasets, bootstrapping and robust standard errors can become more practical due to their ability to handle non-normality and heteroscedasticity. However, it requires careful consideration of the computational resources required and setting appropriate parameters for the method.
VII. Conclusion
A. Recap of key points
Standard error is an important concept in statistical analysis that measures the variability of a statistic due to chance. It allows us to estimate the precision of our estimates and make inferences about populations based on samples. There are several methods for calculating standard error, and common mistakes to avoid. Improving standard error calculations can have a significant impact on statistical analysis, particularly with large datasets.
B. Importance of understanding and accurately calculating standard error
Understanding and accurately calculating standard error is essential for anyone working with data. It allows us to make inferences about populations based on samples and estimate the precision of our estimates. By following the steps outlined in this article, you’ll be well on your way to mastering standard error calculations and improving your data analysis skills.