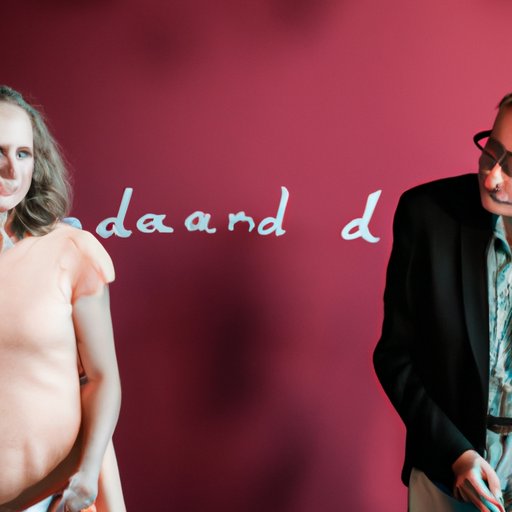
I. Introduction
Standard deviation is a statistical measure that helps in understanding and analyzing the spread, variability, and distribution of data. It provides insights into how much the data points deviate from the average or mean of the dataset. Standard deviation is a crucial metric that has a wide range of applications in various fields, including finance, medicine, education, research, and more.
A. What is Standard Deviation?
Standard deviation, denoted by the symbol σ (sigma), is a statistical measure that represents the amount of deviation or variability within a dataset. It gives an idea of how tightly or loosely the data points are clustered around the mean or average of the dataset. A small standard deviation indicates that the data points are closely packed around the mean, whereas a large standard deviation points to a wider spread of data points from the mean.
B. Why is Standard Deviation important?
Standard deviation is an essential measure in statistics that helps us understand the level of variability or dispersion within a dataset. It aids in identifying and analyzing trends, patterns, and outliers in the data. Standard deviation is also useful in making inferences and conclusions based on the data. Without this statistic, it becomes challenging to make informed decisions or draw accurate conclusions from the data.
C. Who can benefit from understanding Standard Deviation?
Standard deviation is used in various fields, including finance, medicine, education, research, and others. Anyone who deals with data or intends to undertake analysis of any kind can benefit from understanding standard deviation. This includes statisticians, researchers, medical professionals, teachers, students, business analysts, investment managers, and more.
II. Step-by-Step Guide to Calculating Standard Deviation
Here the step-by-step guide to calculating standard deviation:
A. Definition of the Mean
The first step is to calculate the mean or average of the dataset. Add up all the values in the dataset and divide by the number of data points. The resulting number is the mean.
Example:
Data values: 25, 30, 35, 40, 45, 50
Mean = (25+30+35+40+45+50)/6 = 35
B. Finding the Deviations
Next, it’s time to calculate the difference between each data point and the mean value. These differences are known as deviations.
Example:
Data values: 25, 30, 35, 40, 45, 50
Mean = 35
Deviations: -10, -5, 0, 5, 10, 15
C. Squaring the Deviations
Since deviations are measured in units equal to the dataset, they do not cancel out each other. Thus, we square each deviation to make them positive.
Example:
Data values: 25, 30, 35, 40, 45, 50
Mean = 35
Deviations: -10, -5, 0, 5, 10, 15
Squared deviations: 100, 25, 0, 25, 100, 225
D. Calculating the Variance
Variance measures the spread of data from the mean. It is the average of the squared deviations, and it measures the variability around the mean.
Example:
Data values: 25, 30, 35, 40, 45, 50
Mean = 35
Deviations: -10, -5, 0, 5, 10, 15
Squared deviations: 100, 25, 0, 25, 100, 225
Variance = (100+25+0+25+100+225)/6 = 125/3 or 41.67
E. Calculating the Standard Deviation
The final step is to calculate the square root of the variance to find the standard deviation. It is expressed in the same units as the original dataset.
Formula: Standard Deviation (σ) = √Variance
Example:
Data values: 25, 30, 35, 40, 45, 50
Mean = 35
Deviations: -10, -5, 0, 5, 10, 15
Squared deviations: 100, 25, 0, 25, 100, 225
Variance = 125/3 or 41.67
Standard Deviation = √41.67 = 6.46
III. Visualizing Standard Deviation: How to Interpret and Analyze Your Data Spread
Visualizing standard deviation can help in better understanding the distribution of data points and how they are spread out in a dataset. The following are the most common types of data distributions:
A. Normal Distribution
Normal distributions are symmetrical around their mean and are considered as the bell curve. They are characterized by having most data points close to the mean and fewer data points at the tails. In a normal distribution, the standard deviation can be used to understand how widely the data points have spread out.
B. Skewed Distribution
In a skewed distribution, the data is not symmetrical around its mean. It has tails where the data points are more spread out, and it’s characterized as being unbalanced. Using standard deviation on skewed data can provide useful insights into the spread of data points.
C. Bimodal Distribution
In a bimodal distribution, there are two peaks, and the data is not entirely scattered across the mean. It is characterized by having two different sets of data points that could be related but functionally distinct.
D. Interpreting Standard Deviation with Example Distribution Graphs
Below is an example of a distribution with a standard deviation of 1:
Below is an example of a distribution with a standard deviation of 2:
Interpreting graphs like these can make it easier to understand what standard deviation means and how it relates to data points in a dataset.
IV. Tips for Reducing Standard Deviation in Your Dataset for More Accurate Results
Reducing standard deviation in your dataset is vital for more accurate results. The following tips can be useful in reducing the standard deviation in your data:
A. Increase Sample Size
One way to reduce standard deviation is to increase the sample size. The larger the sample size, the more data points are available, and this reduces the effect of outliers.
B. Improve Data Collection Techniques
Proper data collection techniques are essential to ensure accurate and unbiased data. Researchers should ensure consistent methods during data collection, including measurements, timing, and precision.
C. Select a Representative Sample
Choosing a representative sample is essential in data collection. It must be representative of the population being studied, and researchers need to avoid sampling bias.
D. Improve Data Entry Techniques
Improper data entry techniques can lead to data inaccuracies, which influences the standard deviation adversely. Researchers should consider using data entry software to ensure quality and reduce errors.
V. The Impact of Outliers on Standard Deviation and How to Deal With Them
A. Definition of Outliers
Outliers are data points that are unusual, different, or distinct from other data points in the dataset. They can occur due to the measurement error, natural variation, or an extreme event.
B. How Outliers Affect the Standard Deviation
Outliers can have a significant impact on the calculation of standard deviation. If there is an outlier in a dataset, it can cause the standard deviation to be larger than if the outlier did not exist.
C. Identifying Outliers
Outliers can be identified using statistical techniques, including box plots, histograms, and scatter plots. They can also signify incorrect data entry, and researchers can investigate the data for accuracy.
D. Dealing With Outliers
There are different ways to deal with outliers, including removing them from the dataset, reconfirming the data, and including them in a separate dataset. Researchers should consider the implications of excluding or including outliers before making a decision.
VI. Standard Deviation vs. Variance: Understanding the Difference and When to Use Each One
A. Definition of Variance
Variance is another statistical measure that indicates how much the data points are spread out from the mean of the dataset. It is calculated by dividing the sum of squared differences between each data point and the mean by the number of data points.
B. The Relationship between Variance and Standard Deviation
Variance and standard deviation are both measures of dispersion within a dataset. The square of the standard deviation is equal to the variance.
C. Differences Between Variance and Standard Deviation
The primary difference between variance and standard deviation is their values. Standard deviation is expressed in the same units as the dataset, whereas variance is expressed in squared units. Standard deviation is more commonly used than variance, as it is easier to interpret and provides a more precise measure of distribution.
D. When to Use Variance and Standard Deviation
Standard deviation is more commonly used due to its ease of interpretation and precise measure of distribution. However, in some cases, variance may be used when comparing datasets of different sample sizes, as it is more robust to sample size.
VII. Applications of Standard Deviation in Real-World Scenarios
Here the real-world scenarios where standard deviation is commonly used:
A. Finance
Standard deviation is used to measure the risk associated with different investments. It is also used to monitor the fluctuation of stock prices and analyze the performance of mutual funds.
B. Medicine
Standard deviation is used to measure the variation in treatment outcomes within a group of patients. It is used to track the progress of patients and evaluate the effectiveness of medical procedures.
C. Education
Standard deviation is used to measure the overall performance of students in a class. It is also used to track student progress and analyze the effectiveness of educational programs.
D. Research
Standard deviation is used in research to analyze the variability in the results of experiments. It is used in hypothesis testing to describe the level of uncertainty associated with the results.
VIII. Conclusion
Standard deviation is an essential statistical measure that provides valuable insights into the variability and distribution of data. This article has explained standard deviation’s definition, significance, calculation, interpretation and analysis, tips for reducing standard deviation, outliers, and the difference between standard deviation and variance and their applications in real-world scenarios. We hope this beginner-friendly guide has provided you with a solid foundation for understanding standard deviation and how to utilize it in data analysis.