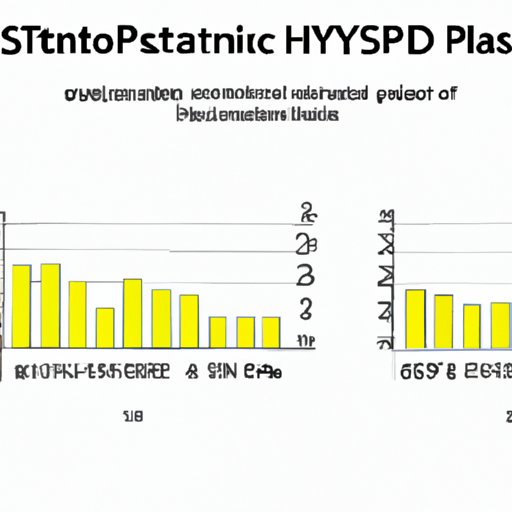
I. Introduction
Test statistic plays a pivotal role in hypothesis testing, which is an essential technique for drawing conclusions about a population based on sample data. In hypothesis testing, we compare a sample statistic to a hypothesized population parameter to determine whether our sample is representative of the population we are interested in studying. Test statistic refers to a summary statistic used in hypothesis testing that measures the degree of evidence against the null hypothesis. In this article, we will provide a beginner-friendly guide to finding test statistic by breaking down the process into comprehensible steps.
II. A Step-by-Step Guide to Finding Test Statistic
Before we get into the steps for finding test statistic, let’s break down what test statistic is and why it is significant in hypothesis testing. Test statistic is a value that is used to decide whether to reject or accept the null hypothesis, which is the hypothesis that there is no significant difference between the sample data and the hypothesized population parameter. The distribution of test statistic is determined by the sampling distribution of the null hypothesis. If the value of the test statistic falls in the rejection region, we reject the null hypothesis; if it falls in the acceptance region, we accept the null hypothesis.
Step A. Determine the null and alternative hypotheses
Before computing the test statistic, we need to articulate clearly what it is we are testing. This is achieved by stating the null hypothesis and the alternative hypothesis. The null hypothesis is the default assumption that there is no significant difference between two or more groups, while the alternative hypothesis is the proposal that there is, in fact, a significant difference.
Example: Suppose we want to test whether the mean weight of apples in two farms is the same. The null hypothesis would be that the mean weight of apples in farm A is equal to the mean weight of apples in farm B, while the alternative hypothesis would be that they are not equal.
Step B. Select the appropriate test and corresponding distribution
The next step is to identify the appropriate test to use and its corresponding distribution. The type of test used depends on the research question, the sample size, and the type of data that is analyzed. The distribution used depends on the type of variables being analyzed, such as normal, t-distribution, chi-square, or F-distribution.
Example: In the above example, we would use a two-sample t-test to compare the means of the two farms.
Step C. Calculate the test statistic using the observed data
Once we identify the appropriate test and distribution, we calculate the test statistic using the observed data. The formula for calculating the test statistic varies depending on the test used. This formula typically involves taking the difference between the sample statistic and the hypothesized population parameter, dividing by the standard deviation, and comparing the result to a distribution.
Example: Using a two-sample t-test, we could compute the test statistic using the following formula:
t = (x̄1 – x̄2) / (s1²/n1 + s2²/n2)^(1/2)
Step D. Determine the p-value and make a decision about rejecting or accepting the null hypothesis
Finally, we determine the p-value and make a decision about rejecting or accepting the null hypothesis. The p-value is the probability of obtaining a value of the test statistic as extreme as the one we calculated if the null hypothesis is true. If the p-value is less than the significance level, we reject the null hypothesis, assuming that there is enough evidence to support the alternative hypothesis. If the p-value is greater than the significance level, we accept the null hypothesis, assuming that there is insufficient evidence to support the alternative hypothesis.
Example: Suppose we set the significance level at 0.05 and get a p-value of 0.02. Since the p-value is less than the significance level, we reject the null hypothesis and conclude that there is a significant difference in the mean weight of apples between the two farms.
III. Understanding the Importance of Test Statistic in Hypothesis Testing
Test statistic is essential in hypothesis testing since it provides a standardized method for examining the difference between sample data and the hypothesized population parameter. It measures the degree of evidence against the null hypothesis and determines whether our sample is representative of the population we want to study.
For example, suppose we want to test whether a new advertisement campaign improves sales. We might create a sample group that is exposed to the ad campaign and a control group that is not exposed. We can then use test statistic to determine whether the difference in sales between the groups is significant or not. If the test statistic is significant, we can conclude that the advertising campaign was effective in generating more sales.
IV. Common Mistakes to Avoid When Finding Test Statistic
Although the process of finding test statistic is relatively straightforward, several common mistakes can occur. Here are a few to look out for:
Mistake 1: Not selecting the correct distribution
Choosing the wrong distribution is one of the most common errors people make when computing test statistic. Selecting the correct distribution depends on the type of data you’re analyzing and the statistical test being used.
Mistake 2: Not standardizing the test statistic correctly
Another common mistake is not standardizing the test statistic properly. Standardization means scaling the data to a standard normal distribution using z-scores. This standardization is necessary for comparing the test statistic with the distribution to determine whether or not to reject the null hypothesis.
Mistake 3: Using the wrong formula for the test statistic
Using the wrong formula for calculating the test statistic can lead to incorrect results. Be sure to check the formula for the specific test you’re using to avoid this mistake.
V. Calculator Apps That Can Help with Calculating Test Statistic
Several calculator applications are available to help people calculate test statistic more easily. Here are three examples:
1. Stat Trek
Stat Trek is a website that provides an online calculator for various statistical tests, including t-tests and z-tests, and probability distributions like normal, t, F, and chi-square. The site provides step-by-step explanations of how each test is done, which is useful for beginners.
2. GraphPad
GraphPad is a software application designed to help individuals in the biostatistics field with statistical analyses. It includes a wide range of features, including calculating test statistics, creating graphs, and automatically determining the appropriate statistical tests to use.
3. Microsoft Excel
Excel is a widely used spreadsheet software that can also be used to perform basic statistical analyses. Features such as the built-in t-test function and the ability to calculate p-values make it a useful tool for calculating test statistics.
VI. Real-World Examples of Test Statistic and Interpreting Results
Real-world examples of test statistics can help us understand their practical implications. Here are a few examples:
1. Medical Research:
Suppose we want to investigate whether a particular medication is effective in reducing blood pressure. We administer the medication to a sample group and a placebo to a control group and compare the mean blood pressures between the two. Using a two-sample t-test, we can calculate the test statistic, which tells us how significant the difference is between the two groups. If the test statistic is significant, we can conclude that the medication is effective in reducing blood pressure.
2. Marketing Research:
Suppose we want to investigate whether a new website design will increase sales. We can compare the conversion rates between the old and new designs using a two-sample z-test. If the test statistic is significant, we can conclude that the new design improves sales and should be implemented.
VII. Conclusion
Test statistic is an integral part of hypothesis testing, which is a fundamental technique for making inferences about a population based on sample data. In this article, we have provided a step-by-step guide to finding test statistic, highlighting its importance in hypothesis testing. We have also emphasized the need to avoid common mistakes while calculating test statistics and suggested some calculator applications that can help make the process easier. Real-world examples have been provided to demonstrate how to use test statistics in practice. We hope this article has provided readers with a comprehensive guide to finding test statistic and its significance in hypothesis testing.