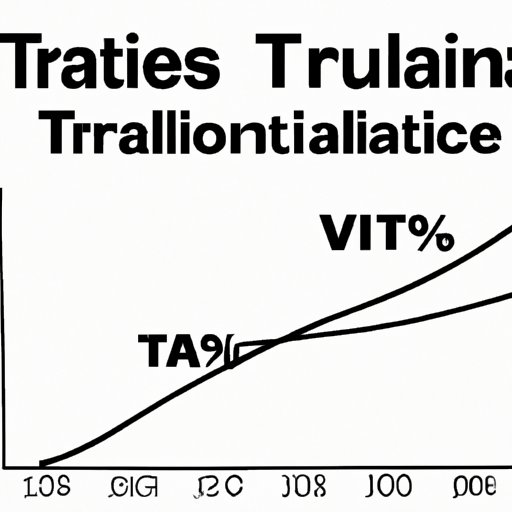
I. Introduction
As a key component of statistical analysis, the critical value is a parameter used to determine the statistical significance of a result. In simple terms, it’s the cutoff point between accepting or rejecting a null hypothesis. Knowing how to find the critical value is crucial for anyone working with statistical tests, as it can greatly impact the accuracy and reliability of their results.
This article aims to provide a comprehensive guide to finding and interpreting critical values. It will cover the steps, common errors, formulas and examples related to critical value determination, as well as tips for quickly finding the critical value.
A. Definition of critical value
The critical value is the value that separates the rejection region from the acceptance region in a statistical test. It is determined by the combination of the significance level and degrees of freedom, and varies depending on the sample size, confidence level, and other factors. When the test statistic falls within the rejection region (ie. below or above the critical value), the null hypothesis is rejected, indicating a significant result.
B. Importance of knowing how to find the critical value
Correctly identifying the critical value is essential for accurate statistical analysis. The improper use of critical values can lead to errors in hypothesis testing, regression analysis, confidence intervals, and other statistical procedures, resulting in invalid conclusions and decisions. On the other hand, mastering critical value determination can improve the quality and reliability of statistical results, allowing researchers to draw valid inferences and make informed decisions.
C. Overview of the article topics
This article will cover the following topics:
- 3 Simple Steps to Find the Critical Value for Any Statistical Test
- Avoid Errors in Statistical Analysis by Mastering Critical Value Determination
- Unlock the Mystery of Critical Value Calculation with these Easy-to-Use Formulas
- A Comprehensive Guide to Finding and Interpreting Critical Values in Statistical Analysis
- Maximize Your Statistics Knowledge: How to Quickly Find the Critical Value and Improve Results
II. 3 Simple Steps to Find the Critical Value for Any Statistical Test
When conducting a statistical test, finding the critical value can be done in 3 simple steps:
A. Step 1: Identify the significance level and degrees of freedom
The significance level is the probability of rejecting a null hypothesis when it is actually true. It is usually set at 0.05 (5%) or 0.01 (1%), but can be adjusted depending on the study design and research question. The degrees of freedom refer to the number of observations that are free to vary in a statistic. It can be calculated using the sample size or the number of groups being compared. Both the significance level and degrees of freedom are needed to determine the critical value.
B. Step 2: Use a critical value table or calculator to find the critical value
Critical value tables or calculators are available for various statistical tests, such as t-test, chi-square test, F-test, and z-test. These tools provide the critical value for a given significance level, degrees of freedom, and two-tailed or one-tailed test. Alternatively, some statistical software programs like SPSS or R can also calculate the critical value automatically.
C. Step 3: Interpret the critical value in relation to the test statistic
After finding the critical value, it is important to compare it to the test statistic calculated from the sample data. If the test statistic is greater than the critical value in the rejection region, the null hypothesis is rejected. On the other hand, if the test statistic falls within the acceptance region, the null hypothesis is not rejected.
III. Avoid Errors in Statistical Analysis by Mastering Critical Value Determination
Although finding the critical value may seem straightforward, there are common errors that can occur in the process. Here are some tips to avoid making mistakes in critical value determination:
A. Common errors in critical value determination
The most common errors in critical value determination include using the wrong significance level or degrees of freedom, selecting the wrong distribution for the test, using a two-tailed test instead of a one-tailed test, and misinterpreting the test statistic. These errors can lead to inaccurate conclusions and false positives or false negatives.
B. Sample size and its effect on critical value determination
The sample size can have a significant impact on critical value determination, particularly with regard to the t-distribution and confidence intervals. As the sample size increases, the degrees of freedom also increase, resulting in smaller critical values and narrower confidence intervals. Therefore, it is important to consider the sample size when selecting the appropriate critical value for a given test.
C. Importance of using the correct critical value for accurate results
Using the correct critical value is crucial for obtaining accurate and reliable statistical results. The incorrect use of critical values can lead to type I or type II errors, affecting the validity and generalizability of the study findings. Therefore, researchers must carefully select the appropriate critical value, taking into account the specific research question, sample size, and other relevant factors.
D. Real-life examples of consequences of incorrect critical value determination
Incorrect critical value determination can have real-life consequences in various fields, such as economics, medicine, and social sciences. For instance, a study investigating the effectiveness of a new drug may falsely conclude that there is a significant difference between the drug and placebo groups, leading to the approval of an ineffective and potentially harmful drug. Conversely, a study analyzing the impact of a public policy may incorrectly reject the null hypothesis, resulting in the rejection or underestimation of the policy’s effects.
IV. Unlock the Mystery of Critical Value Calculation with these Easy-to-Use Formulas
In addition to using critical value tables or calculators, researchers can also calculate critical values using simple formulas. The two most common distributions used in critical value calculation are the standard normal distribution and t-distribution.
A. Explanation of the standard normal distribution and t-distribution
The standard normal distribution is a bell-shaped curve with a mean of 0 and a standard deviation of 1. It is commonly used for tests involving population means, proportions, and correlations, among others. The t-distribution, on the other hand, is a distribution of means for samples smaller than 30, or when the population standard deviation is unknown. It accounts for the increased variability of sample means compared to population means, and has more area in its tails than the standard normal distribution.
B. Formulas for calculating critical values for the standard normal distribution and t-distribution
The formula for calculating the critical value for the standard normal distribution is:
z_crit = ± z_(α/2)
where z_(α/2) is the z-score corresponding to the significance level α divided by 2 (since it is a two-tailed test).
The formula for calculating the critical value for the t-distribution is:
t_crit = ± t_(α/2, df)
where t_(α/2, df) is the t-score corresponding to the significance level α divided by 2 and the degrees of freedom df.
C. Example calculations for both distributions
Suppose we want to calculate the critical value for a two-tailed test with a significance level of 0.05 and a sample size of 20. Using the standard normal distribution, we would find the z-score corresponding to a probability of 2.5% (0.05/2), which is approximately ±1.96. Therefore, the critical value is ±1.96.
Using the t-distribution, we would first calculate the degrees of freedom using the sample size minus 1, which is 19 in this case. Then, we would find the t-score corresponding to a probability of 2.5% and 19 degrees of freedom, which is approximately ±2.093. Therefore, the critical value is ±2.093.
V. A Comprehensive Guide to Finding and Interpreting Critical Values in Statistical Analysis
There are many different types of statistical tests and methods, each with their own specific critical value criteria. Here are some examples of critical value determination for various statistical procedures:
A. Explanation of the different types of statistical tests and their respective critical values
- Hypothesis testing: The critical value is used to determine whether to reject or fail to reject the null hypothesis, based on the test statistic and significance level.
- Confidence intervals: The critical value is used to calculate the margin of error for the confidence interval, based on the sample size and confidence level.
- Regression analysis: The critical value is used to test the significance of the regression coefficients, based on the t-distribution or F-distribution.
- Analysis of variance (ANOVA): The critical value is used to compare the means of multiple groups, based on the F-distribution and degrees of freedom.
- Chi-square test: The critical value is used to test the independence or goodness-of-fit of categorical data, based on the chi-square distribution.
B. Examples of critical value determination for different tests, such as hypothesis testing and regression analysis
Suppose we want to test the hypothesis that the mean weight of a certain product is 50 pounds, based on a sample of 30 products. Using a t-test with a significance level of 0.05, we calculate the t-statistic to be 2.53. The degrees of freedom for the t-distribution are 29. Using a critical value table or calculator, we find the critical value to be ±2.045. Since the t-statistic exceeds the critical value, we reject the null hypothesis and conclude that the mean weight is not 50 pounds.
Suppose we want to perform a multiple regression analysis to predict the sales of a product based on its price and advertising expenditure. We calculate the t-statistic for the regression coefficient of price to be -2.15 and the critical value to be ±1.96, based on a significance level of 0.05 and 25 degrees of freedom. Since the t-statistic falls within the confidence interval defined by the critical value, we fail to reject the null hypothesis and conclude that the regression coefficient of price is not significant.
C. Interpretation of critical values in relation to sample size, confidence level, and other factors.
The interpretation of critical values depends on several factors, such as the sample size, confidence level, and test design. For instance, as the sample size increases, the critical value decreases, indicating a narrower rejection region and a higher likelihood of obtaining a significant result. Similarly, increasing the confidence level widens the confidence interval and increases the critical value, allowing for a greater margin of error. Therefore, researchers must carefully consider the context and purpose of their statistical analysis when interpreting critical values.
VI. Maximize Your Statistics Knowledge: How to Quickly Find the Critical Value and Improve Results
A. Importance of critical value determination in statistical analysis
Mastering critical value determination is essential for anyone working with statistical tests, as it can greatly impact the accuracy and reliability of their results. Whether conducting research, analyzing data, or making decisions based on statistical findings, a solid understanding of critical value determination can improve the quality and validity of the outcomes.
B. Tips for quickly finding the critical value
Here are some tips for quickly finding the critical value:
- Use critical value tables or calculators to avoid manual calculations and reduce errors.
- Double-check the degrees of freedom and significance level to ensure the correct critical value is selected.
- Consider the sample size and confidence level when selecting the appropriate critical value.
- Use statistical software programs like SPSS or R to automate critical value determination and streamline the analysis process.
C. Conclusion: Mastering critical value determination for accurate statistical analysis
By following the steps, formulas, and tips outlined in this article, researchers can improve their understanding of critical values and enhance the accuracy and reliability of their statistical analysis. Whether working with hypothesis testing, regression analysis, or other statistical procedures, mastering critical value determination is an essential skill for any data analyst or researcher. By continuing to learn and apply these concepts in their work, researchers can maximize their statistics knowledge and produce valid and useful results.
VII. Conclusion
In summary, this article has provided a comprehensive guide to finding and interpreting critical values in statistical analysis.