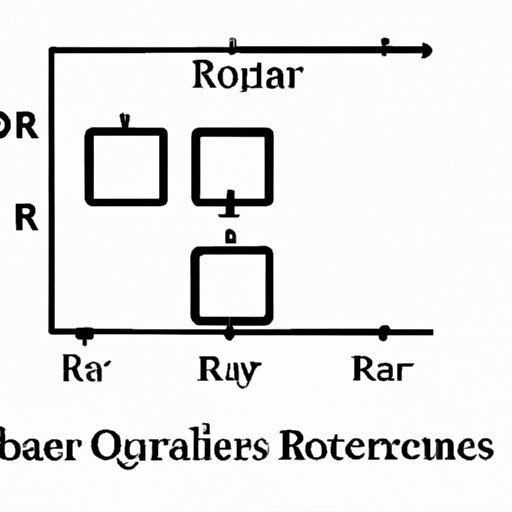
Introduction
The interquartile range, or IQR, is a valuable tool for data analysis. It measures the spread of data and identifies outliers, making it a key component in statistics and scientific research. In this article, we’ll explore in-depth how to find the IQR, why it’s important for data analysis, and how to use it to unlock insights and patterns.
Mastering the Art of IQR: A Step-by-Step Guide
The first step to finding IQR is to sort the data in ascending order. Next, divide the data into quartiles, using the median as the divider between the lower and upper halves of the data set. Then, find the median of the upper and lower halves. Finally, subtract the median of the lower half from the median of the upper half to find the IQR.
Here’s a step-by-step breakdown:
- Sort the data set in ascending order.
- Find the median of the entire data set.
- Divide the data into lower and upper halves, using the median as the divider.
- Find the median of the lower half.
- Find the median of the upper half.
- Subtract the median of the lower half from the median of the upper half to find the IQR.
For example, let’s find the IQR for this data set: 2, 4, 6, 8, 10, 12, 14.
- Sort the data: 2, 4, 6, 8, 10, 12, 14
- Median: 8
- Lower half: 2, 4, 6, 8; Upper half: 10, 12, 14
- Median of lower half: 4
- Median of upper half: 12
- IQR: 12 – 4 = 8
Understanding this basic method for finding the IQR will give you a foundation for exploring more advanced tips and tricks for calculating this valuable data metric.
Calculating IQR Made Simple: Tips and Tricks
Calculating IQR doesn’t have to be a daunting task. Here are some simple tips and tricks to help you find IQR quicker:
Tip 1: Use Technology
Spreadsheet software like Excel can calculate IQR automatically. Simply input your data set, select the data, and use the “QUARTILE.INC” function. This will give you the quartile values necessary to find the IQR.
Tip 2: Visualize Your Data
Use box plots to visualize your data and better understand your quartiles. A box plot displays the median, quartiles, and outliers in a graphical format, making it easier to determine the IQR.
These tips and tricks will help you calculate IQR faster, but understanding the role of IQR in data analysis is equally important.
Unraveling the Mystery of the IQR: A Comprehensive Guide
IQR is a critical component in box plots, which provide a visual representation of the distribution of data. Box plots display the median, upper and lower quartiles, and outliers in a graphical format, allowing for quick and simple data interpretation.
IQR can also be used to identify patterns and trends in data. High IQR values indicate a larger difference between the upper and lower quarters of the data set, which can indicate volatility or variability in the data.
For example, let’s say you are analyzing data on customer spending habits. If the IQR for customer spending is high, this could indicate that customers are spending vastly different amounts of money, indicating a need for further analysis of spending trends. Conversely, a low IQR for customer spending could indicate that spending habits are consistent and less volatile.
Understanding the role of IQR in data analysis is fundamental to unlocking the true potential of this valuable metric.
IQR: What it Is and How to Find It – The Ultimate Guide
This ultimate guide to IQR will provide everything you need to know about this critical data metric, including how to find IQR for different data sets and examples for better understanding. Armed with this knowledge, you’ll be a data analysis pro in no time!
Top Methods to Quickly Calculate IQR for Data Analysis
While the basic method for finding IQR is straightforward, alternative methods can produce quicker and simpler results. The most common method for finding IQR is:
IQR = Q3 – Q1
Where Q1 is the first quartile and Q3 is the third quartile.
Other methods include:
Method 1: Range Method
Calculate the range between the minimum and maximum values in the data set, then divide by 4 to find the width of each quartile. Then, subtract the third quartile from the first quartile to find the IQR.
Method 2: Box Plot Method
Create a box plot of the data set and visually identify the upper and lower quartiles. Then, find the difference between the two quartiles to find the IQR.
Method 3: Standard Deviation Method
Calculate the standard deviation, then multiply by 1.5 to find the upper and lower limits of the IQR. Then, subtract the lower limit from the upper limit to find the IQR.
Using these alternative methods can save time and produce more accurate results, depending on the data set you are analyzing.
Maximizing Data Insights: Using IQR to Unlock Patterns and Trends
One of the most valuable applications of IQR in data analysis is identifying outliers. Outliers can indicate data errors, such as mistyped numbers or mislabeled categories, or can indicate real phenomena that require further analysis. IQR can help you identify these outliers and determine how to proceed with your data analysis.
IQR is also valuable for detecting patterns and trends in data. By identifying larger differences between the upper and lower quartiles, you can uncover insights that might otherwise go unnoticed.
For example, let’s say you are analyzing sales data for a clothing retailer. If the IQR for sales data is high for a particular product category, this could indicate that certain styles or product lines are selling better than others, indicating opportunities for further analysis and investment.
Finding the IQR Like a Pro: Expert Strategies for Accurate Results
While the basic method for finding IQR is straightforward, there are several factors to consider when using this valuable data metric. For example, different data sets may have different distributions, which can affect how you calculate IQR. Understanding these factors is essential for accurate and effective data analysis.
Here are some expert strategies for finding IQR accurately:
Strategy 1: Pay Attention to Data Distribution
Understanding how the data is distributed can affect how you calculate IQR. For example, if the data set is heavily right-skewed, the IQR will be larger than if the data set is normally distributed.
Strategy 2: Use Appropriate Quartile Definitions
There are different definitions of quartiles that can affect how you calculate IQR. Understanding which quartile definition to use for your data set is critical for accurate results.
Strategy 3: Be Aware of Data Outliers
Data outliers can affect the accuracy of IQR, which is why it’s important to identify and deal with them appropriately. Generally, outliers are defined as data points that are more than 1.5 times the IQR away from the nearest quartile.
By employing these strategies, you can find IQR accurately and use it to unlock patterns and trends in your data with confidence.
Conclusion
Understanding IQR is fundamental to effective data analysis. By mastering the art of IQR, you can quickly and accurately calculate this valuable data metric and use it to unlock insights and patterns in your data.
Whether you’re a beginner or an expert in data analysis, the implications of IQR for pattern recognition, detection of outliers, and other applications make it an essential metric to understand. With this ultimate guide, you’ll be equipped to master IQR and make the most of your data analysis.