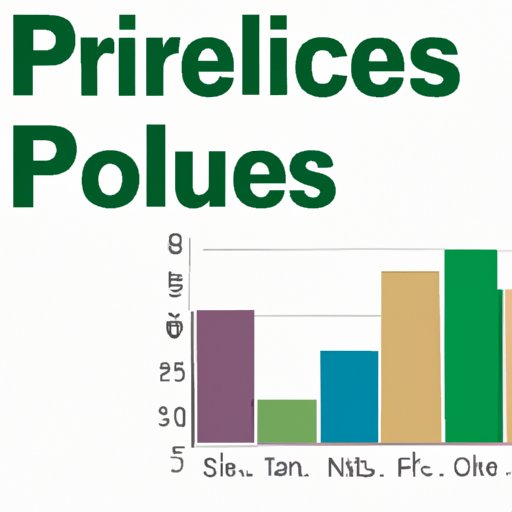
I. Introduction
As a beginner researcher, trying to make sense of the statistical data can be overwhelming. One standard component of data analysis that you might have heard of is p values. P values are an essential tool in scientific research that enables researchers to test hypotheses. This guide aims to provide a thorough understanding of p values, the significance of p values, and how to interpret them correctly. By the end of this article, you will come away with a comprehensive guide to the art of interpreting p values.
II. Mastering the Art of P Values: A Comprehensive Guide
What are p values and how do they work?
P value is a statistical measure that determines the likelihood of the obtained result happening by chance. In simpler terms, it is the probability that the data you collected could have occurred if the null hypothesis were true. Null hypothesis is the assumption that there is no relationship between the variables studied. When the p-value is small, it implies that the observed results are unlikely to happen by chance, leading us to reject the null hypothesis.
The relationship between p values and statistical significance
P value and statistical significance go hand in hand. The convention in scientific research is that when the p-value is less than 0.05, the result is considered statistically significant. This threshold of 0.05 indicates that there is less than a 5% chance of the result being a fluke or happening at random. In contrast, a p-value greater than 0.05 implies that there is a high chance that your results would happen by chance.
Types of p values
There are different types of p values, including one-tailed and two-tailed p values. A one-tailed test predicts in advance the direction of the expected relationship between the variables under analysis. It is useful when we have a specific a priori hypothesis. On the other hand, a two-tailed test has no predetermined direction of the hypothesis and is more appropriate in exploratory research. Reporting the one-tailed test as a two-tailed test is a common mistake that must be avoided.
III. Demystifying P Values: Understanding Statistical Significance
Definition of statistical significance
Statistical significance is the probability of the observed result being due to a real and genuine relationship between the variables under analysis. It is a crucial concept in research since it helps in deciding whether to accept or reject the null hypothesis.
How p values determine statistical significance
The relationship between p value and statistical significance is inverse. A lower p value signifies higher statistical significance. The threshold for statistical significance is typically set at p < 0.05. With this threshold, we can conclude that the results are statistically significant if there is sufficient evidence to infer that the data could not have occurred by chance.
The difference between statistical and practical significance
While statistical significance is all about mathematical calculations and probability, practical significance is real-world or practical usefulness. Even if our results are statistically significant, it might not necessarily have practical significance. For example, a study might find that a new drug reduces cancer by 1%, statistically significant, but not practically significant.
IV. Why P Values are Important: A Step-by-Step Guide to Interpretation
Standard interpretation of p values
Interpreting p-values is a critical aspect of statistical analysis. When the p-value is less than 0.05, we reject the null hypothesis, and the observed result is statistically significant. When the p-value is greater than 0.05, we accept the null hypothesis, and the result is not statistically significant. Whenever interpreting p values, it is important to consider the context of the research question and the study design.
How to interpret low and high p values
A low p value indicates strong evidence against the null hypothesis, while a high p value indicates weak evidence against null hypothesis. Different fields tend to use different thresholds for interpreting p-values as statistically significant. Therefore, it is essential to consult the literature of your field to know what is commonly used.
Examples of p value interpretation
Suppose we are conducting a study to determine if there is a difference in IQ scores between two groups of students. If our p-value is 0.01, we can conclude that the difference we have observed between the two groups is statistically significant. This suggests that the groups have different mean IQ scores. On the other hand, if our p-value is 0.1, this would suggest that there is not enough evidence to support the hypothesis that the two groups’ mean IQ scores are different.
V. Interpreting P Values: How to Avoid Common Mistakes
The dangers of misinterpreting p values
Misinterpreting p values can lead to several issues, including false positives, failing to detect genuine relationships, and misdiagnosing statistical significance. Therefore, it is important to understand and interpret p values correctly to make appropriate conclusions.
Common mistakes in interpreting p values and how to avoid them
One common mistake researchers make is to use a p-value without considering its context or study design. Another common mistake is to think that rejecting the null hypothesis means that the alternative hypothesis is automatically true, which is not the case. A third common mistake is using a p-value threshold of 0.05 for all studies indiscriminately. To avoid these errors, it is crucial to have a good understanding of statistical concepts, the research question, and the study design.
Best practices for p value interpretation
The following best practices will help in interpreting p values effectively: always consider the context of the research question and design, use an appropriate threshold for statistical significance, be cautious when interpreting borderline p-values, and be aware of the effects of multiple testing to avoid increasing the risk of false-positive results.
VI. Making Sense of P Values: A Practical Guide for Researchers
Importance of p values in scientific research
If you are a researcher, understanding and interpreting p values is essential in validating your research questions and testing hypotheses. P values allow you to know if observed results arose by chance or were real and genuine relationships between the variables. They also help you make better decisions, be critical, and distinguish significant results from non-significant ones.
How to report and communicate p values effectively
When reporting p values, it is crucial to include relevant contextual information for the reader. This includes information about the study design, sample size, and statistical tests used. It is also important to report the effect size to offer additional insights into the strength and magnitude of the observed relationships.
Real-life examples of p value interpretation in research
One example of p value interpretation in research is a study conducted by a team of scientists to assess if a new drug reduces the risk of heart diseases. The results showed a p value of 0.02, which suggests that the new drug was effective in reducing the risk of heart diseases. Another example is a study in which researchers investigated the relationship between weight gain during pregnancy and newborn body weight. The p value was 0.9, illustrating that there was no significant relationship between weight gain during pregnancy and newborn body weight.
VII. The Importance of P Values in Scientific Research: A Beginner’s Guide
Basic concepts of scientific research and hypothesis testing
If you are new to scientific research, it is essential to understand the basics of research methodology, hypothesis testing, and statistical analysis. A hypothesis is a tentative statement that a researcher makes to explain the observed phenomenon. To test the hypothesis, researchers collect data and analyze it using statistical tools such as p value.
The role of p values in hypothesis testing
P values play a crucial role in hypothesis testing by providing a quantitative measure of statistical evidence against the null hypothesis. This evidence helps researchers to make conclusions and draw valid inferences.
The importance of understanding p values for beginner researchers
For beginner researchers, understanding p values is essential in increasing their knowledge and expertise in research methodology and statistical analysis. Besides, p values help beginner researchers to develop critical thinking skills, evaluate the quality of research, and make evidence-based conclusions.
VIII. Beyond the Basics: Advanced Techniques for Interpreting P Values
Advanced statistical techniques for p value interpretation
Advanced statistical techniques for p value interpretation include Bayesian analysis, effect size estimations, and confidence intervals. Bayesian analysis considers prior beliefs about the relationship under analysis and combines them with new data to update their beliefs. Effect size estimations allow researchers to obtain additional information about the magnitude of the relationship between variables. Confidence intervals are range estimates surrounding an observed effect size that provides an idea of where the true value lies.
How to handle multiple comparisons and p-value adjustments
Multiple testing occurs when a researcher tests many hypotheses on the same data set. With multiple comparisons, the risk of committing a Type I error increases, which leads to false-positive results. To mitigate this risk, researchers typically use methods such as Bonferroni correction, Benjamini-Hochberg correction, or permutation tests, among others.
The future of p values in scientific research
P values continue to evolve with the changing scientific landscape. Emerging trends such as open science, data sharing, and machine learning will influence the way p values are reported, analyzed and interpreted. Researchers can expect an increase in the use of innovative statistical tools and methods that will improve their work’s accuracy, validity, and reliability.
IX. Conclusion
In conclusion, understanding p values is an essential tool for researchers when testing hypotheses and making evidence-based conclusions. The art of interpreting p values requires careful consideration of the context and design of the research question. By applying the techniques and strategies outlined in this comprehensive guide, researchers can gain a good understanding and be confident in interpreting p values and statistics better. Start interpreting p values with these techniques, and you will elevate your research and become a better data analyst.