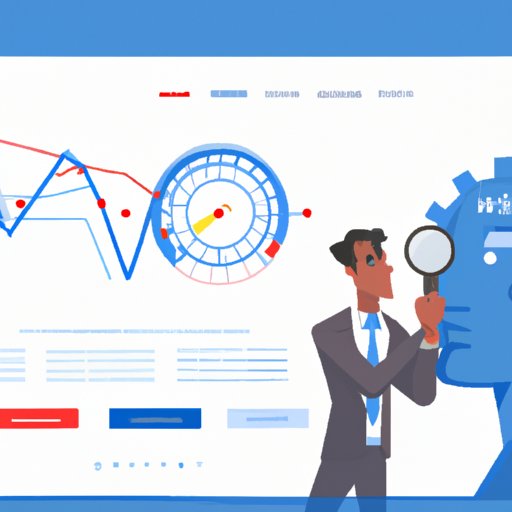
Introduction
Predictive analysis is the use of statistical models and machine learning algorithms to predict future outcomes. It is an advanced form of data analysis that uses historical data to identify patterns and relationships, which can then be used to make predictions about future events and trends.
The importance of predictive analysis lies in its ability to help businesses and organizations make more informed decisions and take proactive measures to improve their performance. By accurately predicting future outcomes, organizations can anticipate and plan for potential challenges and opportunities, which can help them stay ahead of their competitors.
Examples of how predictive analysis has been used in different fields and industries include predicting customer behavior and preferences, forecasting financial trends, optimizing operations, and detecting fraud.
While there are many benefits to predictive analysis, it is not without its limitations. One of the main limitations is that it relies heavily on historical data, which may not always be an accurate predictor of future events. Additionally, predictive analysis algorithms can be complex and difficult to interpret, which can limit their usefulness in some applications.
Guide to Predictive Analysis for Beginners
If you’re new to predictive analysis, it can seem daunting. However, the key concepts and techniques can be explained in simple terms to help beginners understand the basics.
The most common statistical techniques used in predictive analysis are regression analysis and decision trees. Regression analysis involves using historical data to identify patterns and relationships, which can then be used to make predictions about future events. Decision trees are graphical representations of the possible outcomes of a decision, and they can be used to identify the best decision based on historical data.
Advanced algorithms such as neural networks and genetic algorithms can also be used for predictive analysis. Neural networks are used to identify complex patterns in large sets of data, while genetic algorithms are used to find the optimal solution to complex problems.
Ethical and Social Implications of Predictive Analysis
The use of predictive analysis has raised ethical and social implications, particularly in areas such as law enforcement, healthcare, and finance. There is a concern that the use of algorithms in decision-making processes can lead to bias and discrimination, as well as questions about how data privacy is being protected.
One of the biggest issues with the use of predictive analysis is the potential for bias. Algorithms can be trained on biased data, which can lead to discriminatory outcomes. Additionally, there can be a lack of transparency in how algorithms are used, which can limit the ability to detect and correct bias.
To address these concerns, some organizations have developed best practices for the use of predictive analysis, such as requiring the use of diverse data sets and providing transparency in the decision-making process. Additionally, there is a need for ongoing research and collaboration to identify potential biases and develop solutions to mitigate them.
Overview of Tools and Technologies Used in Predictive Analysis
There are many different tools and technologies used in predictive analysis, including popular software packages, programming languages, and machine learning frameworks. Some of the most popular software packages for predictive analysis include SAS, IBM SPSS, and R. Python and R are two of the most popular programming languages used for predictive analysis.
Machine learning frameworks, such as TensorFlow and Scikit-learn, are also commonly used for predictive analysis. These frameworks provide a set of tools and algorithms that can be used to develop predictive models and analyze data.
The choice of tools and technologies will depend largely on the specific needs and requirements of the organization. Each tool or technology has its own strengths and weaknesses, and it is important to carefully evaluate them before making a decision.
Case Study: Predictive Analysis in Action
To illustrate how predictive analysis can be used to solve real-world problems, let’s look at a few examples.
Predicting customer behavior is one area where predictive analysis can be particularly useful. For example, a company may use predictive analysis to identify customers who are at risk of cancelling their subscription or leaving for a competitor. This can help the company take proactive measures to retain these customers, such as offering discounts or personalized offers.
Forecasting financial trends is another area where predictive analysis can be used. For example, a bank may use predictive analysis to forecast loan defaults or identify patterns in customer spending behavior. This can help the bank make more informed decisions about lending and risk management.
Optimizing operations is another area where predictive analysis can be used. For example, a manufacturing company may use predictive analysis to identify areas where production can be optimized, such as identifying bottlenecks in the supply chain or predicting maintenance needs for equipment.
Results from predictive analysis can be presented in a clear and visually engaging way, such as through dashboards or interactive reports. This can help decision-makers quickly and easily identify trends and make informed decisions based on the results.
Conclusion
Predictive analysis is a powerful tool that can be used to make accurate predictions and forecasts about future events and trends based on historical data. While there are many benefits to using predictive analysis, there are also ethical and social implications that must be considered.
As predictive analysis continues to evolve, it is important for organizations to stay up-to-date with the latest tools and technologies, as well as best practices for ensuring that algorithms are transparent and free from bias. By doing so, organizations can make more informed decisions and stay ahead of their competitors in today’s data-driven world.
If you’re interested in learning more about predictive analysis, we encourage you to explore the many resources available and consider taking a course or certification program to develop your skills.