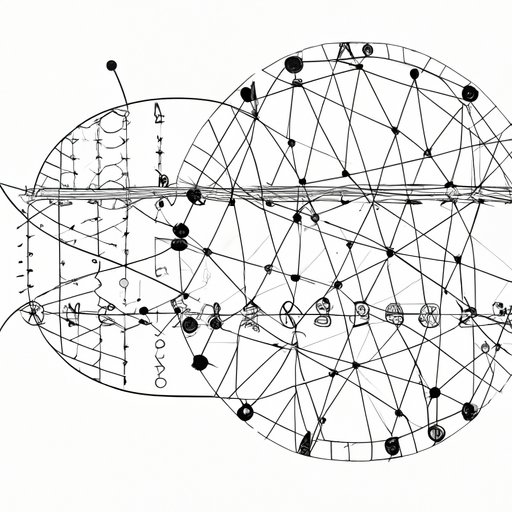
Introduction
Principal component analysis (PCA) refers to a statistical process that converts complex datasets into simpler structures. In other words, PCA is an algorithm that identifies patterns in data, allowing data analysts to represent these patterns visually and numerically. Understanding PCA is crucial for effective data analysis, especially in big data projects that involve processing vast amounts of information.
Principal Component Analysis 101: Understanding the Basics
At the core of PCA is the concept of a principal component. A principal component is a linear combination of original variables that captures the most significant variability in data. It is a new variable that summarizes the information present in the original dataset, allowing analysts to visualize patterns and relationships in the data that would otherwise be hard to discern. PCA achieves this by identifying the most significant axes in the dataset, rotating and transforming data onto these axes to generate principal components.
The benefits of PCA in data analysis are significant and far-reaching. For instance, PCA allows data analysts to reduce data dimensionality while preserving data variation, making it easier to visualize and interpret data. Moreover, PCA helps to identify underlying structures within data and can be used to filter out noise effectively.
The basic steps involved in PCA include: data scaling, correlation matrix calculation, eigenvalue decomposition of the correlation matrix, and variable weighting based on correlation coefficients.
Unraveling the Mystery Behind Principal Component Analysis
Despite its immense benefits, PCA remains a relatively misunderstood concept in data analysis, with many misconceptions. For instance, some analysts believe that PCA is only useful when data is Gaussian distributed. However, PCA works on all types of data with a correlation structure since its primary goal is determining the most significant variation in a dataset.
PCA also differs from other data analysis methods, such as clustering. Clustering seeks to group similar observations based on a distance metric. PCA categorizes variables based on their significance in explaining the variation in a dataset.
Real-world examples of PCA’s successful application include face recognition, bioinformatics, finance, and image compression algorithms.
Applaud Your Data Analysis with Principal Component Analysis
Choosing PCA over other data analysis methods has several advantages, which include dimensionality reduction, quick identification of variable importance, and data visualization. PCA is also useful in exploratory data analysis since it provides a quick and efficient way of identifying data patterns without prior knowledge of the data.
PCA can also save time in data analysis, especially when dealing with complex datasets, by providing a holistic view of the data. This eliminates the need for specialized analysis techniques, reducing analysis time while delivering relevant insights.
Diving Deep into Principal Component Analysis: Techniques, Challenges, & Applications
Advanced techniques have been developed for PCA, such as nonlinear PCA and sparse PCA. Nonlinear PCA works by projecting data onto a low-dimensional space while still preserving nonlinear structures. Sparse PCA achieves dimensionality reduction by selecting only the most important variables in a dataset, making it more efficient for analysis.
There are also common challenges in implementing PCA, such as dealing with missing data and determining the number of principal components appropriate for a dataset. However, these challenges can be overcome with careful consideration and effective data pre-processing.
Real-world applications of PCA abound in various industries, with examples ranging from gene expression analysis in bioinformatics, anomaly detection in cybersecurity, and customer segmentation in marketing.
Simplifying Complex Data Structures with Principal Component Analysis
PCA simplifies complex data structures by identifying significant patterns within datasets. This allows analysts to eliminate irrelevant variables and condense data into understandable visualizations. PCA is particularly useful in large datasets, which can be challenging to analyze due to the sheer volume of data.
Real-world examples of using PCA to simplify complex data structures include demography-based analysis, where PCA is used to establish relationships between demographic factors and lifestyle choices, and finance, where PCA determines the correlation between stock prices and macroeconomic indicators.
Conclusion
PCA is a crucial concept in data analysis, with immense benefits in simplifying data structures and improving insights. Despite its many advantages, PCA is still fraught with misconceptions and challenges, making it critical for analysts to have a clear understanding of its underlying principles and techniques. With its many applications and benefits, PCA is a tool that all data analysts should consider incorporating into their data analysis processes to improve data comprehension.